The Transformative Potential of Machine Learning Programs in Business
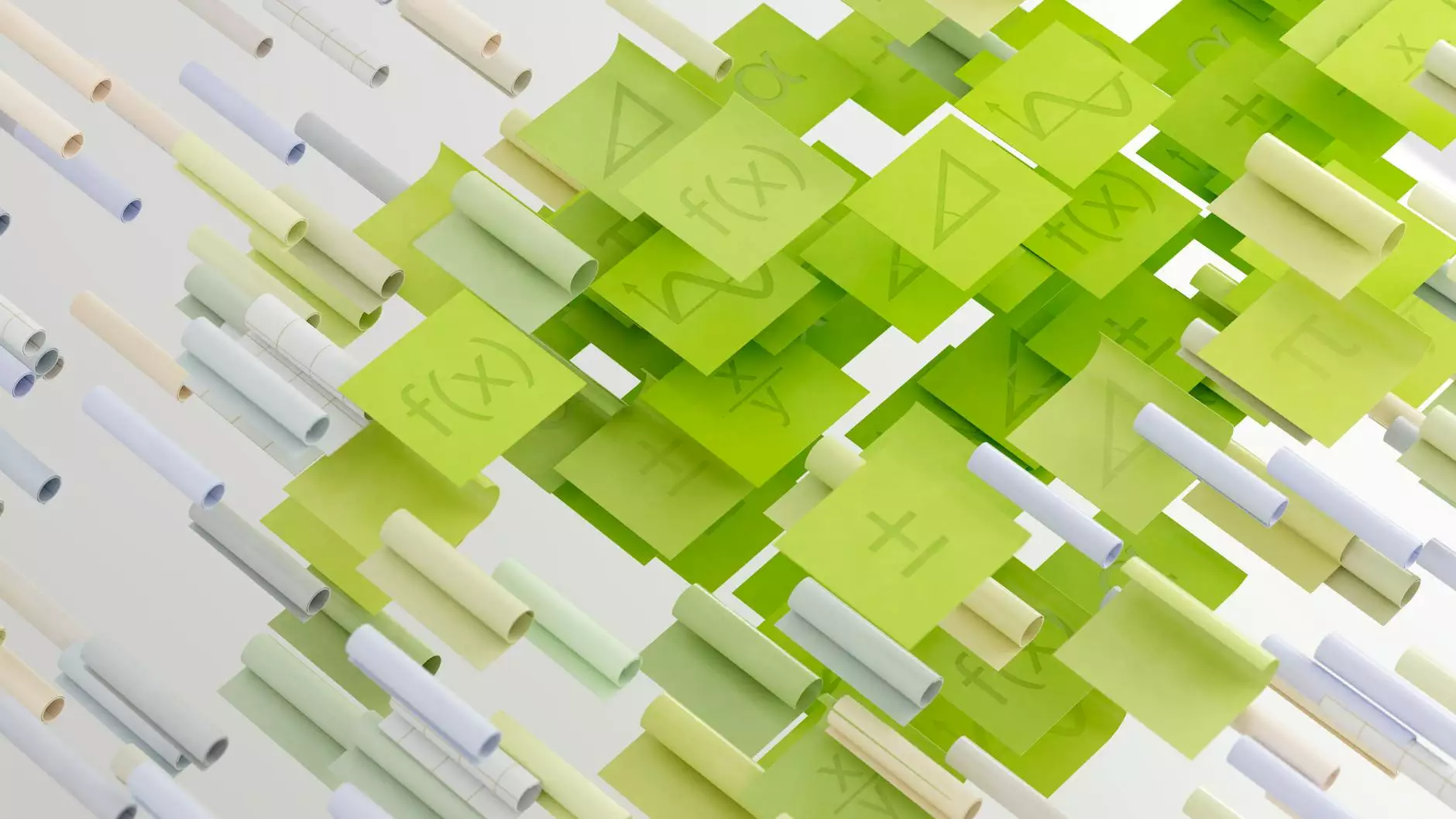
In the rapidly evolving landscape of business today, organizations are increasingly turning to technology to enhance their operations and gain a competitive edge. Among the most significant technological advancements is the rise of machine learning (ML). This article delves into the programs for machine learning that are revolutionizing various business processes, specifically in the realms of content management services, business process automation services, and data governance systems. By understanding how to leverage these tools effectively, businesses can unlock unprecedented growth and efficiency.
Understanding Machine Learning
Machine learning is a branch of artificial intelligence that allows systems to learn from data and improve over time without being explicitly programmed. Instead of relying on human intervention for every decision, ML algorithms can analyze vast datasets, identify patterns, and make predictions. This capability makes them indispensable for modern businesses striving to enhance productivity, optimize operations, and deliver more personalized experiences to their customers.
Programs for Machine Learning: Key Types and Tools
When considering the integration of programs for machine learning, it is essential to recognize the diverse range of tools and platforms available. Here are some of the most prominent categories:
- Data Preparation Tools: These programs help businesses clean and organize their data for analysis. Notable examples include Talend, Apache Nifi, and Alteryx.
- Machine Learning Frameworks: Frameworks like TensorFlow, PyTorch, and scikit-learn provide foundational building blocks for developing ML models.
- Automated Machine Learning (AutoML) Tools: Tools such as H2O.ai and Google Cloud AutoML simplify the process of creating and deploying ML models.
- Big Data Technologies: Programs like Apache Spark facilitate the processing and analysis of large data sets, which is crucial for effective machine learning.
Machine Learning in Content Management Services
Content management is at the heart of many organizations, and the integration of programs for machine learning can significantly enhance this area. Here’s how:
1. Enhanced User Personalization
Machine learning algorithms can analyze user behavior and preferences to deliver a tailored content experience. By utilizing programs for machine learning, businesses can ensure that their customers receive content that is most relevant to them, thus increasing engagement and retention rates.
2. Improved Search Functionality
Machine learning can optimize search engines within content management systems (CMS). Advanced algorithms can understand user queries better and provide more accurate search results. For instance, natural language processing (NLP) features allow the system to grasp context and intent, leading to improved search capabilities.
3. Automated Content Tagging
ML programs can automate the process of tagging and categorizing content, using algorithms to understand the nature of the content and the appropriate labels. This capability allows for more efficient content management, making it easier for businesses to maintain and access their resources.
Revolutionizing Business Process Automation Services with Machine Learning
Business process automation (BPA) aims to streamline operations and tasks to increase efficiency. Programs for machine learning play a crucial role in achieving these objectives:
1. Predictive Analytics for Decision Making
Machine learning algorithms can analyze historical data to make predictions about future trends and performance. This information enables businesses to make informed decisions, optimizing resource allocation and operational strategies.
2. Intelligent Process Automation
Combining robotic process automation (RPA) with machine learning can yield intelligent process automation solutions. While traditional RPA handles repetitive tasks, machine learning adds an element of decision-making capability, allowing for more complex processes while reducing the need for human oversight.
3. Continuous Improvement
Machine learning programs offer the ability to learn from each interaction. This leads to a cycle of continuous improvement in business processes, allowing the organization to adapt to changing market conditions and operational demands more swiftly.
Strengthening Data Governance Systems with Machine Learning
In today’s data-driven world, robust data governance is crucial for compliance and effective management of sensitive information. Programs for machine learning play a key role in enhancing data governance systems:
1. Automated Data Classification
Machine learning algorithms can automate the classification of data into categories based on its content and sensitivity. This automation not only speeds up the process but also helps organizations maintain compliance by ensuring that sensitive data is properly managed.
2. Anomaly Detection
Machine learning can be utilized to develop systems that monitor datasets in real-time, identifying anomalies that could indicate a breach or error. This proactive approach helps organizations mitigate risks and ensures the integrity of their data.
3. Enhancing Reporting and Audit Trails
With the integration of ML programs, reporting systems can be enhanced to provide more in-depth insights into data usage and governance practices. This enhancement allows for more effective auditing and transparency in data handling procedures.
Real-world Applications of Machine Learning in Business
Numerous organizations across different industries are leveraging programs for machine learning to achieve their business goals. Here are a few noteworthy examples:
- Retail: Retail companies use machine learning for inventory management and personalized marketing, leading to better customer experiences and increased sales.
- Finance: Financial institutions implement ML algorithms for fraud detection, risk assessment, and customer service chatbots, enhancing productivity and customer satisfaction.
- Healthcare: In healthcare, machine learning assists in predictive analytics for patient care, diagnosis support systems, and efficient administrative processes.
- Manufacturing: Manufacturers utilize ML for predictive maintenance, improving equipment reliability and reducing downtime.
Challenges and Considerations in Implementing Machine Learning
While the benefits of programs for machine learning are substantial, businesses must also address several challenges during implementation:
1. Data Quality and Availability
Machine learning is heavily reliant on high-quality data. Organizations must ensure that their data is accurate, complete, and accessible; otherwise, the effectiveness of ML models may be compromised.
2. Talent Gap
There is a significant demand for skilled professionals who can implement and manage machine learning technologies. Companies may face challenges in finding and retaining talent with the necessary skills in data science and ML.
3. Ethical Considerations
The use of machine learning raises ethical questions related to data privacy and algorithmic bias. Organizations need to establish policies that regard the ethical implications of their ML applications, ensuring fairness and transparency.
Conclusion
The future of business is intertwined with the advancements in technology, particularly through programs for machine learning. By integrating machine learning into their operations, companies can enhance content management services, automate business processes, and strengthen data governance systems. As the landscape continues to evolve, those who embrace machine learning will undoubtedly position themselves at the forefront of their industries, reaping the rewards of efficiency, innovation, and growth.
Intalio.com stands ready to assist organizations looking to implement these transformative machine learning solutions, providing expertise in content management, business process automation, and data governance systems. The time to act is now; the future of business depends on it.